大学院生で国際会議やジャーナル投稿のために英語論文を書く場合に、これだけは最低限やっておいてほしいことをまとめたいと思います。
初めて英語論文を書く人は、どう書いて良いのかよくわからないかと思います。よく分からない状態で書き始めたら、間違いなく微妙な論文が出来上がります。
どんなに素晴らしい研究内容でも、書き方が微妙だと査読に落ちてしまいます。逆にいえば、内容が微妙でも、書き方がわかりやすいと通ったりもします。
「研究が遂行できる能力」と同じくらい「論文の書く力」は重要です!
なので論文の書き方の基本は必ず体系的に学んでおいて下さい!(特に博士課程の人は!)
こちらの記事にも書き方TIPSを書いているのでよかったら読んで下さい。
まずは本を読む!授業を受ける!
通りやすくなる書き方のテクニックはもちろんありますが、これはあくまで基礎が出来ているからこそ効果があります。
まずは書き方の「基礎」を体系的に学んでください。
そもそもバッドにボールが当たらないのにホームランの打ち方を学んでもホームランは打てません(笑)
近道はありませんので、まずは「基礎」!
面倒臭いとは思いますが、ここでやるかやらないかで今後の人生大きく左右すると思います。
特に研究者やグローバルで活躍したいと思う人は。
出来て当たり前、出来ないとどんどん時代に取り残されます。
ぜひ歯を食いしばって苦手な英語に立ち向かって下さい。
学生さんであれば「Academic Writing」の講義を受講して下さい。
受講できない人は本で勉強しましょう。
論文の書き方の基本を学ぶ上で参考にした書籍をいくつか紹介しますので、よかったら参考にしてみてください。
どの参考書でも似たような内容なので、どれか1冊をきっちり抑えれば大丈夫です。
オンラインでアカデミックライティングを学べる講座もありますので、本を読むのが面倒臭い人は受けてみても良いかもしれません。1000円くらいで受けられます。
上手い人の論文を読み込んで、構造を真似する!
基礎を固めた上で、上手い人の論文を熟読するのは本当に大事です。
論文の章構成・構造は、上手い人の論文をそのまま真似しちゃって下さい。
構造を真似しても剽窃にはなりません。
上手い人の書き方を真似れば良い論文に近づくのは必然です。
パナソニックの創業者の松下幸之助も「まずは真似る」と仰ってました。
IMRADを遵守するのは当然です。
IMRADという言葉が分からない人はスタートラインに立ってませんので、まずは基礎を学んで下さい。
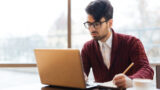
真似するところはもう少し細かいところです。
ロボット分野で有名な論文誌(IEEE Transactions on Robotics)にOpen Accessとして掲載されている論文のIntroductionを例にとって見てみましょう。
State estimation is undoubtedly the most fundamental module for a wide range of applications, such as robotic navigation, autonomous driving, virtual reality, and augmented reality (AR). Approaches that use only a monocular camera have gained significant interests in the field due to their small size, low-cost, and easy hardware setup [1]–[5]. However, monocular vision-only systems are incapable of recovering the metric scale, therefore, limiting their usage in real-world robotic applications. Recently, we have seen a growing trend of assisting the monocular vision system with a low-cost inertial measurement unit (IMU). The primary advantage of this monocular visualinertial system (VINS) is to observe the metric scale, as well as roll and pitch angles. This enables navigation tasks that require metric state estimations. In addition, the integration of IMU measurements can dramatically improve the motion-tracking performance by bridging the gap between losses of visual tracks due to illumination change, textureless area, or motion blur. The monocular VINS is not only widely available on ground robots and drones, but also practicable on mobile devices. It has great advantages in size, weight and power consumption for self and environmental perception.
However, several issues affect the usage of monocular VINS. The first one is rigorous initialization. Due to the lack of direct distance measurements, it is difficult to directly fuse the monocular visual structure with inertial measurements. Also recognizing the fact that VINSs are highly nonlinear, we see significant challenges in terms of estimator initialization. In most cases, the system should be launched from a known stationary position and moved slowly and carefully at the beginning, which limits its usage in practice. Another issue is that the long-term drift is unavoidable for visual-inertial odometry (VIO). In order to eliminate the drift, loop detection, relocalization, and global optimization has to be developed. Except for these critical issues, the demand for map saving and reuse is growing.
To address all these issues, we propose VINS-Mono, a robust and versatile monocular visual-inertial state estimator, which is the combination and extension of our three previous works [6]–[8]. VINS-Mono contains following features:
1) robust initialization procedure that is able to bootstrap the system from unknown initial states;
2) tightly coupled, optimization-based monocular VIO with camera–IMU extrinsic calibration and IMU bias correction;
3) online relocalization and four degrees-of-freedom (DOF) global pose graph optimization;
4) pose graph reuse that can save, load, and merge multiple local pose graphs.
Among these features, robust initialization, relocalization, and pose graph reuse are our technical contributions, which come from our previous works [6]–[8]. Engineering contributions include open-source system integration, real-time demonstration for drone navigation, and mobile applications. The whole system has been successfully applied to small-scale AR scenarios, medium-scale drone navigation, and large-scale state estimation tasks, as shown in Fig. 1.
T. Qin et al., “VINS-Mono: A Robust and Versatile Monocular Visual-Inertial State Estimator”, IEEE TRANSACTIONS ON ROBOTICS, VOL. 34, NO. 4, AUGUST 2018
まず第1パラグラフを見てみましょう。
- State estimation is undoubtedly the most fundamental module for a wide range of applications, such as robotic navigation, autonomous driving, virtual reality, and augmented reality (AR). Approaches that use only a monocular camera have gained significant interests in the field due to their small size, low-cost, and easy hardware setup [1]–[5].
- まず自分の研究分野の技術の大枠の背景・特徴を話します。
- However, monocular vision-only systems are incapable of recovering the metric scale, therefore, limiting their usage in real-world robotic applications.
- 次にその問題点・課題を指摘します。
- Recently, we have seen a growing trend of assisting the monocular vision system with a low-cost inertial measurement unit (IMU). The primary advantage of this monocular visualinertial system (VINS) is to observe the metric scale, as well as roll and pitch angles. This enables navigation tasks that require metric state estimations. In addition, the integration of IMU measurements can dramatically improve the motion-tracking performance by bridging the gap between losses of visual tracks due to illumination change, textureless area, or motion blur. The monocular VINS is not only widely available on ground robots and drones, but also practicable on mobile devices. It has great advantages in size, weight and power consumption for self and environmental perception.
- 最近の流行り・特徴について紹介します。
次に第2パラグラフです。
- However, several issues affect the usage of monocular VINS.
- 最近の流行りにもまだ問題点・課題があることを話します。
- The first one is rigorous initialization. Due to the lack of direct distance measurements, it is difficult to directly fuse the monocular visual structure with inertial measurements. Also recognizing the fact that VINSs are highly nonlinear, we see significant challenges in terms of estimator initialization. In most cases, the system should be launched from a known stationary position and moved slowly and carefully at the beginning, which limits its usage in practice.
- 1つ目の問題点について。
- Another issue is that the long-term drift is unavoidable for visual-inertial odometry (VIO). In order to eliminate the drift, loop detection, relocalization, and global optimization has to be developed. Except for these critical issues, the demand for map saving and reuse is growing.
- 2つ目の問題点について。
そして第3パラグラフです。
- To address all these issues, we propose VINS-Mono, a robust and versatile monocular visual-inertial state estimator, which is the combination and extension of our three previous works [6]–[8].
- 上述した問題に対して、今回自分達はどう解決するか紹介します。
- VINS-Mono contains following features: 1) robust initialization procedure that is able to bootstrap the system from unknown initial states; 2) tightly coupled, optimization-based monocular VIO with camera–IMU extrinsic calibration and IMU bias correction; 3) online relocalization and four degrees-of-freedom (DOF) global pose graph optimization; 4) pose graph reuse that can save, load, and merge multiple local pose graphs.
- 解決方法をもう少し詳しく話します。
- Among these features, robust initialization, relocalization, and pose graph reuse are our technical contributions, which come from our previous works [6]–[8]. Engineering contributions include open-source system integration, real-time demonstration for drone navigation, and mobile applications. The whole system has been successfully applied to small-scale AR scenarios, medium-scale drone navigation, and large-scale state estimation tasks, as shown in Fig. 1.
- 今回の論文の新規性・貢献は何なのかアピールします。
どうでしょうか、非常にシンプルで分かりやすい構成かなと思います。
- 大枠の背景
- 問題提起
- 最新の動向
- 技術課題
- 研究目的・新規性
良い論文を書く人は大体このフレームワークに沿って書いてます。
Method・Resultsは分野によって書き方は違ってくるので割愛しますが、上手い人の書き方は分かりやすい構造になっているはずなので熟読してみて下さい。
また赤線でハイライトしたところは、非常によく使われるフレーズで、言いたいことが書かれているサインとして使います。
However(またはMeanwhile、しばしばbut)が使う時は、その後に大事な主張を述べるのが基本です。
なのでHoweverの多用は主張が分かりづらくなってしまうので避けましょう。
To address all these issuesもよく使われるフレーズで、これから自分の解決手法を述べるサインになりますので、レビューワーも手法の概要を知るためにこのようなフレーズが文章内にないか探します。
あとContribution(またはNovelty/Originality)というワードも必ず入れてほしいです。これでこの論文の新規性が何なのかバシッと掴めます。
余計なことは書かず、シンプルで論理的な繋がりがあるセンテンスに
卒論を書き終えた学生さんが、論文投稿する際によく見る傾向として、余計な情報が非常に多い。
それはおそらく卒論を書く上で文字数稼ぎのために本来なくても伝わる文章が付け加えられて内容が伝わり図らくなっているからです。
論文には過不足のない情報が求められます。そして1文1文、論理的に繋がっていないといけません。
先ほど引用した論文のIntroductionの第1パラグラフを改めて日本語で見てみましょう。
- 状態推定は、ロボットナビゲーション、自律走行、バーチャルリアリティ、拡張現実感(AR)など、様々なアプリケーションにおいて最も基本的なモジュールであることは間違いありません。
- 単眼カメラのみを用いたアプローチは、小型・低コストでハードウェアのセットアップが容易であることから、この分野で大きな関心を集めています。
- しかし、単眼カメラのみを用いたシステムでは、メートル単位での復元ができないため、実世界のロボットアプリケーションでの利用は限定的です。
- 近年、低コストな慣性計測ユニット(IMU)を用いて単眼式視覚システムを補助する動きが活発化しています。
- この単眼式視覚慣性システム(VINS)の主な利点は、ロール角やピッチ角と同様にメートル単位での観測が可能なことです。
- これにより、メートル法による状態推定が必要なナビゲーションタスクが可能になります。
- また、IMUの計測値を統合することで、照明の変化、テクスチャのない領域、あるいはモーションブラーによる視覚トラックの損失間のギャップを埋めることにより、モーショントラッキングパフォーマンスを劇的に向上させることができます。
- 単眼のVINSは、地上ロボットやドローンに広く搭載されているだけでなく、モバイル機器でも実用化されています。
- また自己認識や環境認識において、サイズ、重量、消費電力の面で大きな優位性を持っています。
専門分野でなくても、流れはスッと理解できるのではないでしょうか。
いきなり文章として書くのではなく、上記のように一回リスト化してみて、それぞれのセンテンスが論理的につながっているか、余計な文章を書いてないかをチェックすることをお勧めします。
これはパラグラフ・ライティングという技法で、分かりやすい文章を書くうえで必須のスキルです。
こちらのサイトにパラグラフ・ライティングに関してまとまっておりますので一読いただければと思います。
最新のツールを使いこなして正しい文章を
下記の記事でもまとめておりますが、最近はもう色々便利な翻訳・添削ツールがあります。
なので、英語が苦手な人は、まずは日本語で洗練化された文章を書いて下さい。
ここでの洗練化というのは英訳しやすい日本語で書くということです。
日本語はある程度省略されても文章が成立してしまいます。
例えば、
「研究目的をロボットの開発とする」
という文章はよく見ると「主語」がありません。
英語に訳しやすい日本語に直すと、
「本研究の目的は(S)ロボットの(O)開発である(V)」
となります。
日本語的には一見大丈夫そうな文章も、英文化すると「あれ?この文章情報足りなくない?」ということはよくあります。
ぜひ「この日本語は英語に直すことができるのか?」という視点でご自身の文章をチェックしてみて下さい。
そして洗練化された日本語さえ完成すればあとはDeepLに突っ込めば精度の高い英文が生成されるはずです!
これから英語論文を書き始める人、なかなか書くのが上手くならない人にとって少しでも参考になれば嬉しいです!
コメント